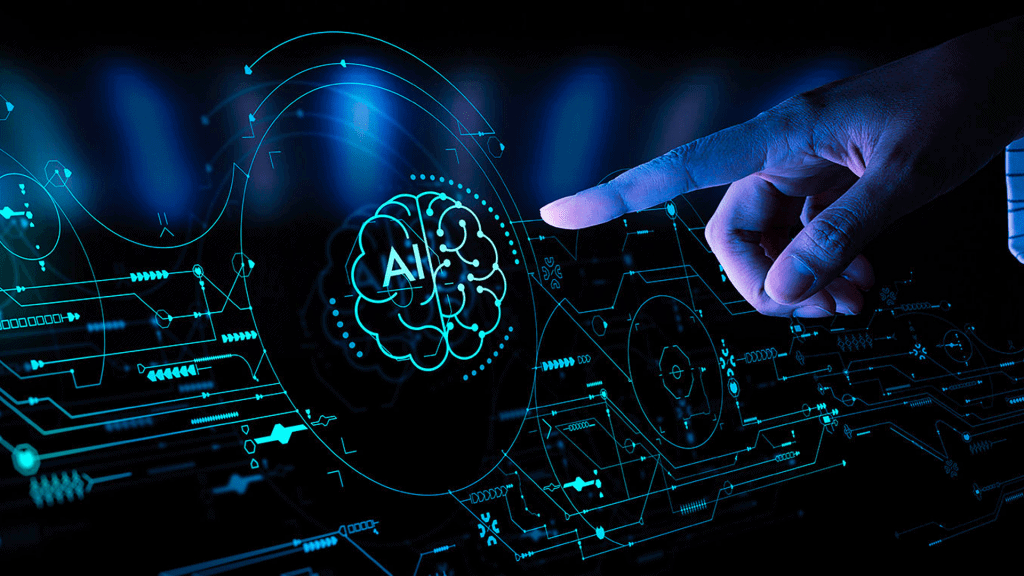
How Cybersecurity Enhancing Defense Against Emerging Threats?
Cybersecurity plays a crucial role in enhancing defense against emerging threats through various measures and technologies:
- Threat Intelligence Sharing: Organizations actively share information about emerging threats, attack vectors, and vulnerabilities. This collaborative approach allows for early identification and response to new and evolving threats.
- Advanced Threat Detection: Cybersecurity systems employ advanced technologies like machine learning, artificial intelligence (AI), and behavior analytics to detect and identify emerging threats. These systems analyze vast amounts of data in real-time to recognize patterns, anomalies, and indicators of compromise.
- Endpoint Protection: Endpoint security solutions are continuously improving to defend against emerging threats. They employ techniques such as next-generation antivirus, behavioral analysis, and sandboxing to detect and mitigate malware, ransomware, and other malicious activities.
- Cloud Security: As organizations increasingly adopt cloud services, cybersecurity measures are enhanced to protect cloud infrastructure, data, and applications. Robust access controls, encryption, and continuous monitoring help safeguard against emerging cloud-based threats.
- Network Segmentation: Implementing network segmentation divides networks into smaller, isolated segments. This practice limits lateral movement for attackers and contains potential breaches, minimizing the impact of emerging threats.
- User Authentication and Access Controls: Strong user authentication mechanisms, such as multi-factor authentication (MFA) and privileged access management (PAM), are critical in defending against emerging threats. These measures ensure that only authorized individuals have access to sensitive systems and data.
- Security Awareness Training: Educating employees about cybersecurity best practices is vital. Training programs raise awareness about emerging threats, social engineering attacks, and phishing attempts, empowering employees to recognize and respond appropriately.
- Incident Response and Threat Hunting: Organizations establish robust incident response plans and proactive threat hunting capabilities. This allows for the early detection, containment, and remediation of emerging threats, minimizing their impact on systems and data.
- Patch Management: Regular patching and updates of software and systems are essential to address vulnerabilities that can be exploited by emerging threats. Effective patch management practices ensure that systems are up to date and protected against known vulnerabilities.
- Collaboration and Information Sharing: Organizations collaborate with cybersecurity vendors, industry alliances, and government agencies to share threat intelligence and best practices. These collaborations foster a collective defense approach, enabling the rapid dissemination of information and coordinated responses to emerging threats.
It’s important to note that cybersecurity is an ongoing process, and organizations must continuously adapt and evolve their defenses to stay ahead of emerging threats. By leveraging advanced technologies, fostering a security-aware culture, and collaborating with industry partners, organizations can enhance their defense capabilities against emerging threats.
How AI Enhancing Defense Against Emerging Threats?
AI is revolutionizing defense against emerging threats in cybersecurity through various ways:
- Advanced Threat Detection: AI-powered systems can analyze vast amounts of data in real-time, including network logs, user behavior, and system files, to detect patterns and anomalies that may indicate potential threats. Machine learning algorithms can learn from historical data and adapt to identify new and evolving threats, allowing organizations to detect and prevent attacks more effectively.
- Anomaly Detection: AI can establish baseline behaviors for users, systems, and networks, continuously monitoring for deviations from these patterns. By leveraging machine learning algorithms, AI systems can identify anomalies that may indicate security breaches or malicious activities, enabling early detection and response to emerging threats.
- Behavioral Analytics: AI algorithms can analyze user behavior to identify suspicious or abnormal activities, both within an organization and externally. By detecting unusual patterns, AI systems can generate alerts for further investigation, empowering organizations to proactively address emerging threats, such as insider threats or unauthorized access attempts.
- Threat Intelligence and Analysis: AI can automate the analysis of large volumes of threat intelligence data, including information on known threats, vulnerabilities, and indicators of compromise. By continuously processing and correlating this data, AI systems can identify potential emerging threats, providing valuable insights to security teams.
- Incident Response and Mitigation: AI can assist in incident response by automating certain tasks like containment, isolation, and remediation. By analyzing attack patterns and historical data, AI systems can suggest appropriate response measures, helping organizations mitigate the impact of security incidents in a timely manner.
- Vulnerability Management: AI can aid in identifying vulnerabilities in networks, systems, and applications. By analyzing code, configurations, and system logs, AI algorithms can pinpoint weaknesses that could be exploited by attackers. This proactive vulnerability management approach allows organizations to address security gaps before they are exploited by emerging threats.
- Phishing and Fraud Detection: AI techniques, such as natural language processing and machine vision, can be employed to recognize patterns in emails, websites, or other communication channels commonly used for phishing attacks. AI systems can identify suspicious content, URLs, or visual cues that indicate fraudulent activity, helping organizations defend against emerging phishing threats.
- Adversarial Attack Detection: AI can be utilized to identify adversarial attacks aimed at subverting or evading security measures. AI systems can detect patterns or anomalies in data that indicate attempts to manipulate or deceive AI models, allowing organizations to strengthen their defenses against emerging attack techniques.
By harnessing the power of AI, organizations can significantly enhance their ability to detect, respond to, and mitigate emerging threats in real-time. It’s important to note that while AI is a valuable tool, it should be used in conjunction with human expertise and comprehensive security practices for effective defense against emerging threats.
AI Enhances Network Monitoring and Threat Detection
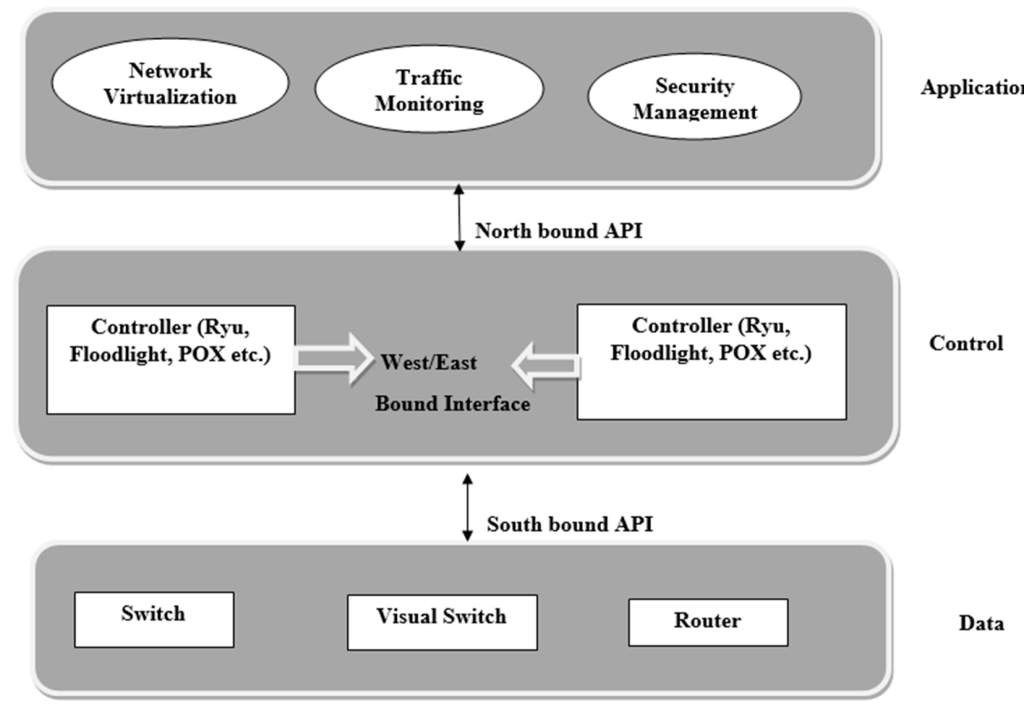
AI brings significant advancements to network monitoring and threat detection, enhancing the capabilities of security systems. Here’s how AI enhances these areas:
- Real-time Network Monitoring: AI-powered systems continuously monitor network traffic in real-time. By analyzing vast amounts of data, AI can identify patterns, anomalies, and potential threats, enabling early detection and response.
- Automated Threat Detection: AI enables automated detection of threats by analyzing network behavior, communication patterns, and data flows. It can identify deviations from normal behavior and detect suspicious activities indicating ongoing attacks or intrusions.
- Anomaly Detection: AI algorithms establish baselines of normal network behavior and detect anomalies that deviate from those patterns. This helps identify new and previously unknown threats, including zero-day attacks or sophisticated intrusion attempts.
- Behavioral Analytics: AI analyzes user behavior, device interactions, and access patterns to identify abnormal activities or indicators of compromised accounts. By detecting suspicious behavior, AI systems can raise alerts or trigger additional security measures for investigation.
- Intelligent Log Analysis: AI can analyze system logs, security event data, and other relevant sources to identify security incidents or indicators of compromise. By correlating and analyzing log data in real-time, AI systems can detect and respond to potential threats more effectively.
- Predictive Analytics: AI leverages historical and real-time data to predict potential security incidents or vulnerabilities. By analyzing patterns and trends, AI systems provide insights that enable proactive measures to mitigate risks before they materialize.
- Deep Packet Inspection: AI performs deep packet inspection, analyzing network traffic at a granular level. This allows the detection of malicious code, suspicious payloads, or network anomalies that traditional rule-based systems might miss.
- Threat Intelligence Integration: AI ingests and analyzes large volumes of threat intelligence data from various sources. By integrating threat intelligence feeds, AI systems stay updated on the latest known threats, attack techniques, and indicators of compromise, enhancing their threat detection capabilities.
- Reduced False Positives: AI systems learn from historical data and feedback to improve the accuracy of threat detection, reducing false positives. This helps security teams focus their efforts on genuine threats, improving operational efficiency.
- Continuous Learning and Adaptation: AI systems continuously learn from new data, evolving threat landscapes, and feedback from security analysts. This enables them to adapt and improve their threat detection capabilities over time, staying ahead of emerging threats.
By leveraging AI technologies, organizations can enhance their network monitoring and threat detection capabilities. AI systems automate processes, provide real-time insights, and augment the capabilities of security teams, resulting in stronger cybersecurity defenses.
AI Performs Predictive Analysis
Predictive analysis is a task performed by AI systems that utilize advanced algorithms and machine learning techniques to analyze data and generate predictions about future outcomes. Here’s how AI enables predictive analysis:
- Data Collection and Integration: AI systems gather and integrate data from various sources, including structured and unstructured data, internal and external datasets, and real-time streams. This data can consist of historical records, sensor data, customer information, market trends, and more.
- Data Preprocessing and Feature Engineering: AI algorithms preprocess and transform the data to prepare it for analysis. This includes tasks such as data cleaning, normalization, feature selection, and extraction. Feature engineering involves identifying relevant variables and creating new features that capture meaningful patterns and relationships.
- Model Training and Selection: AI models are trained using historical data, which includes input features and corresponding outcomes. During the training process, AI algorithms learn from the data to identify patterns, correlations, and dependencies. Various machine learning algorithms, such as regression, decision trees, neural networks, and ensemble methods, can be employed for training.
- Prediction Generation: Once the AI model is trained, it can generate predictions by inputting new or unseen data. The model applies the learned patterns and relationships to make predictions about future outcomes or events. The predictions can take the form of binary (yes/no), categorical (class labels), or continuous (numeric values).
- Model Evaluation and Refinement: AI models are evaluated based on performance metrics such as accuracy, precision, recall, or mean squared error. If the model’s performance is not satisfactory, it can be refined by adjusting hyperparameters, adding more training data, or employing different algorithms.
- Continuous Learning and Updating: AI models can be designed to continually learn and update themselves as new data becomes available. This allows the model to adapt to changing patterns and improve the accuracy of its predictions over time.
- Decision Support: Predictive analysis generated by AI provides valuable insights and supports decision-making processes. For instance, it can assist organizations in forecasting demand, anticipating customer behavior, optimizing resource allocation, detecting anomalies or fraud, and identifying emerging trends or risks.
- Scenario Modeling and What-If Analysis: AI models can be used to simulate different scenarios and perform what-if analysis. By manipulating input variables, decision-makers can evaluate the potential impact of different strategies or interventions and make informed decisions based on the predicted outcomes.
- Forecasting and Trend Analysis: AI can analyze historical data to identify trends, patterns, and seasonality. This enables organizations to make predictions about future trends, market conditions, or customer preferences, aiding in planning and strategic decision-making.
- Risk Assessment and Mitigation: AI can assess risks by analyzing historical data and identifying factors that contribute to specific outcomes. This enables organizations to identify potential risks and take proactive measures to mitigate them.
The predictive analysis capabilities of AI empower organizations to leverage data-driven insights for informed decision-making, future event anticipation, and business process optimization. By utilizing AI to identify patterns and relationships within data, organizations can gain a competitive edge and make more accurate predictions across various domains.
AI Augments Human Security Analysts
AI serves as a valuable tool to augment human security analysts, enhancing their capabilities and improving overall cybersecurity operations. Here’s how AI enhances the work of security analysts:
- Threat Intelligence Analysis: AI systems gather and analyze extensive volumes of threat intelligence data from diverse sources, such as security feeds, forums, and social media. By automatically processing and correlating this information, AI provides security analysts with valuable insights, enabling them to stay up-to-date on emerging threats and make well-informed decisions.
- Automated Data Analysis: AI algorithms excel at analyzing large quantities of security-related data, such as logs, network traffic, and system events, more rapidly and accurately than humans. By automating data analysis, AI helps security analysts identify patterns, detect anomalies, and uncover potential security breaches that may go unnoticed with manual analysis.
- Alert Prioritization and Triage: AI aids in triaging and prioritizing security alerts based on their severity and likelihood of being legitimate threats. By leveraging machine learning algorithms on historical data, AI systems learn from past analyst decisions and offer recommendations to help analysts focus their attention on critical alerts, saving time and improving efficiency.
- Pattern Recognition and Anomaly Detection: AI excels at recognizing patterns and identifying anomalies in data. By analyzing network traffic, user behavior, and system logs, AI systems can detect unusual activities and behaviors that may indicate potential security breaches. This empowers security analysts to promptly investigate and respond to emerging threats.
- Malware Analysis: AI-based systems can automate the analysis of malware samples, examining their behavior, code, and characteristics. By employing machine learning and behavioral analysis techniques, AI can identify new and unknown malware variants, enabling security analysts to develop countermeasures and protect systems against emerging threats.
- Incident Response Support: AI can assist security analysts during incident response by providing real-time guidance and recommendations. By analyzing incident data, AI systems can suggest remediation steps, containment strategies, and incident handling procedures, helping security analysts respond effectively and efficiently to security incidents.
- Contextual Insights: AI systems can provide security analysts with contextual information by aggregating and correlating data from various sources. By integrating threat intelligence, vulnerability data, and network information, AI offers a comprehensive view of the security landscape, enabling analysts to better understand the impact and scope of security incidents.
- Predictive Analytics: AI leverages historical data and machine learning algorithms to predict potential security threats and vulnerabilities. By analyzing patterns and trends, AI systems can forecast potential risks, enabling security analysts to take proactive measures and allocate resources effectively to mitigate emerging threats.
- User and Entity Behavior Analytics: AI algorithms can analyze user behavior, access patterns, and interactions with systems to detect suspicious activities or insider threats. By flagging unusual behaviors, AI systems help security analysts investigate potential security breaches and identify compromised accounts or systems.
- Enhanced Decision Support: AI systems provide security analysts with additional insights and recommendations based on their analysis of data. By considering a broader range of factors and patterns, AI augments human decision-making, enabling analysts to make more informed choices during complex and time-sensitive security operations.
It’s important to recognize that while AI augments the work of security analysts, human expertise, critical thinking, and contextual understanding remain crucial for effective cybersecurity. The collaboration between AI and human analysts creates a powerful combination to combat emerging threats and strengthen overall defense capabilities.
AI and Cybersecurity: Enhancing Defense Against Emerging Threats
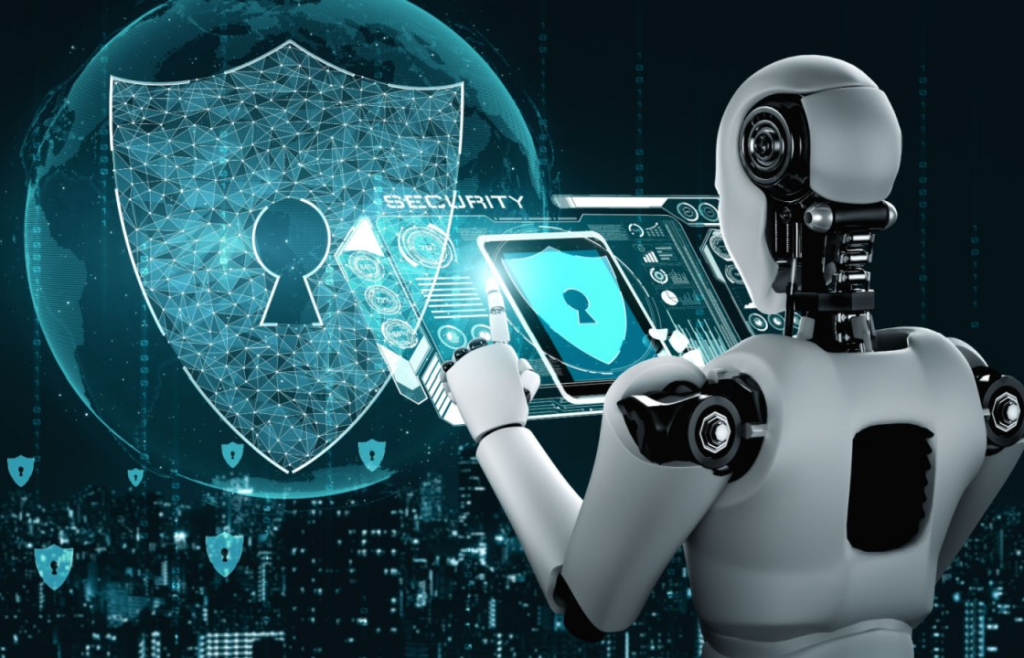
AI and cybersecurity work together to enhance defense against emerging threats, leveraging advanced technologies and capabilities to strengthen security measures. Here are some key ways in which AI enhances defense against emerging threats:
- Advanced Threat Detection: AI-powered systems analyze diverse data sources such as network logs, system events, and user behavior to identify patterns and anomalies that may indicate potential security breaches or emerging threats. Machine learning algorithms enable AI to detect unknown or evolving attack techniques that traditional security tools might miss.
- Real-time Monitoring and Incident Response: AI enables real-time monitoring of systems and networks, continuously analyzing incoming data for signs of suspicious activity. It promptly identifies and alerts security teams to potential threats, allowing for swift response and mitigation. Additionally, AI systems can automate incident response actions, such as isolating affected systems, blocking malicious traffic, or initiating remediation measures.
- Behavioral Analysis: AI algorithms learn normal patterns of user and system behavior within an organization. By continuously monitoring and analyzing behaviors, AI can identify deviations or anomalies that may indicate malicious activity or compromised accounts. This aids in detecting emerging threats, such as insider threats or targeted attacks, which exhibit abnormal behavior patterns.
- Threat Intelligence and Predictive Analysis: AI processes large volumes of threat intelligence data from various sources, including public feeds and research reports. By aggregating and correlating this information, AI systems can identify emerging threats, vulnerabilities, and attack trends. Predictive analysis assists organizations in anticipating future attack vectors and implementing proactive defense strategies.
- Vulnerability Management: AI helps identify and prioritize vulnerabilities within an organization’s systems and infrastructure. It analyzes system configurations, code, and patch levels to pinpoint potential weaknesses. This enables security teams to focus their efforts on addressing critical vulnerabilities and mitigating emerging threats effectively.
- User Authentication and Access Control: AI enhances user authentication mechanisms by considering multiple factors, such as user behavior, device information, and contextual data. Machine learning algorithms enable AI to detect unusual login patterns or suspicious activity, enhancing security measures and preventing unauthorized access.
- Malware Detection and Analysis: AI plays a crucial role in malware detection and analysis. It identifies known malware signatures and behavioral patterns, as well as detects new and unknown malware variants based on their behavior. AI-powered systems automate the analysis of malware samples, classify them, and extract indicators of compromise (IOCs) for faster response and mitigation.
- Security Automation and Orchestration: AI automates security processes, reducing manual effort and response time. It can handle routine security tasks such as log analysis, incident triaging, and security policy enforcement. This allows security analysts to focus on complex threats and strategic security initiatives.
- Adaptive and Self-Learning Defenses: AI systems adapt and learn from evolving threats and attack techniques. Continuous analysis of new data and feedback enables AI algorithms to improve detection capabilities and adjust defense strategies accordingly. This adaptability ensures organizations remain resilient against emerging threats.
- Enhanced Decision Support: AI provides security analysts with contextual information, insights, and recommendations based on comprehensive data analysis. This empowers analysts to make informed decisions, prioritize response efforts, and allocate resources effectively when facing emerging threats.
The collaboration between AI and cybersecurity strengthens defense capabilities, enabling organizations to proactively identify and respond to emerging threats in real-time. It’s important to note that while AI enhances defense, human expertise, domain knowledge, and ethical considerations remain essential for effective cybersecurity.
Thanks,
Leave a Reply
You must be logged in to post a comment.