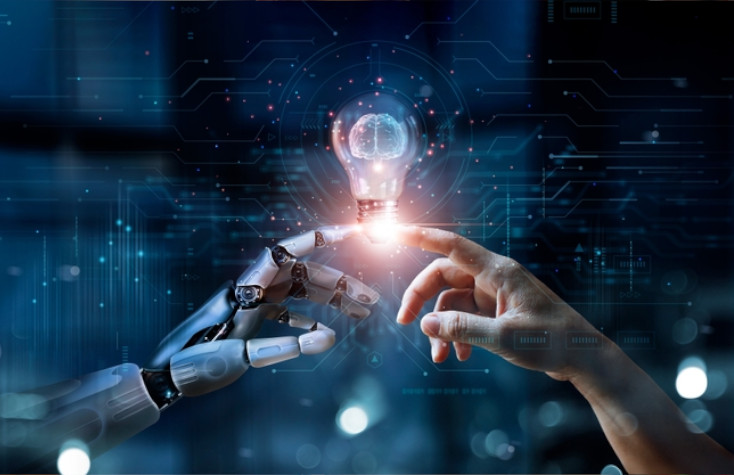
Introduction
In today’s fast-paced world, effective decision-making and strategic planning rely heavily on our ability to anticipate and prepare for the future. With the ever-increasing complexity and abundance of data, harnessing the power of artificial intelligence (AI) tools for future forecasting has become paramount. By embracing AI’s capabilities, we gain valuable insights, make well-informed choices, and confidently navigate uncertain terrains.
AI, including machine learning and predictive analytics, has revolutionized data processing and interpretation. Its advanced algorithms enable the identification of hidden patterns, correlations, and trends within vast datasets, surpassing human capabilities in speed and efficiency. As a result, AI has unlocked new possibilities and enhanced traditional forecasting methods.
This blog delves into the captivating world of AI tools for future forecasting, exploring their applications across various industries and their transformative impact on decision-making processes. Additionally, we will examine different techniques employed in future forecasting, shedding light on AI’s capacity to predict outcomes with remarkable precision. However, alongside the potential, we will also address challenges and ethical considerations linked to AI adoption in forecasting.
As businesses and societies continue to evolve, staying abreast of technological advancements is crucial. Join us on this journey of discovery, as we unveil the immense potential and profound influence of harnessing AI tools for future forecasting. Together, let’s explore how AI can shape a more prepared and promising future for all.
Highlight of the significance of future forecasting in decision-making and strategic planning.
Future forecasting is of paramount importance in decision-making and strategic planning, providing invaluable insights that can profoundly influence the success of individuals, businesses, and organizations. Here are some key reasons why future forecasting holds such significance:
- Anticipating Emerging Trends and Opportunities: Future forecasting enables decision-makers to identify emerging trends and potential opportunities in the market. By understanding what the future may hold, businesses can proactively position themselves to capitalize on these upcoming trends, gaining a competitive advantage.
- Mitigating Risks: Alongside recognizing opportunities, future forecasting helps identify potential risks and challenges. Armed with this knowledge, organizations can develop risk management strategies to minimize adverse impacts and ensure business continuity.
- Efficient Resource Allocation: Strategic planning involves allocating resources effectively. Future forecasting allows decision-makers to make data-driven choices about where to invest time, money, and effort, optimizing resource allocation for maximum returns.
- Informed Decision-making: When decision-makers have access to accurate predictions and insights, their choices become more informed and less reliant on guesswork. This leads to more effective and confident decision-making processes.
- Facilitating Long-Term Planning: Strategic planning often involves making decisions that will shape the organization’s trajectory for years to come. Future forecasting provides a foundation for long-term planning, ensuring that actions align with future goals and visions.
- Agility and Adaptability: With future forecasting, organizations can become more agile and adaptable in responding to changes in the market or external environment. This enables them to adjust their strategies and tactics promptly, maintaining competitiveness.
- Driving Innovation and Product Development: Understanding future trends and customer demands empowers businesses to innovate and develop products or services that cater to evolving needs, ensuring continued relevance in the market.
- Resource Efficiency: Future forecasting allows organizations to optimize resource allocation, reducing wasteful spending and improving overall operational efficiency.
- Performance Evaluation: Comparing actual outcomes with forecasted ones aids in evaluating the effectiveness of strategies and identifying areas that require improvement.
- Gaining a Competitive Advantage: Organizations that effectively utilize future forecasting gain a competitive edge over those that rely solely on historical data or intuition. It empowers them to anticipate changes and respond proactively, positioning themselves ahead in dynamic markets.
In conclusion, future forecasting serves as a powerful tool, empowering decision-makers and strategic planners with invaluable insights into the future business landscape. By leveraging data-driven predictions, organizations can make well-informed decisions, enhance their strategic planning, and confidently navigate uncertainty, ultimately laying the foundation for sustainable growth and success.
Understanding AI Tools for Future Forecasting.
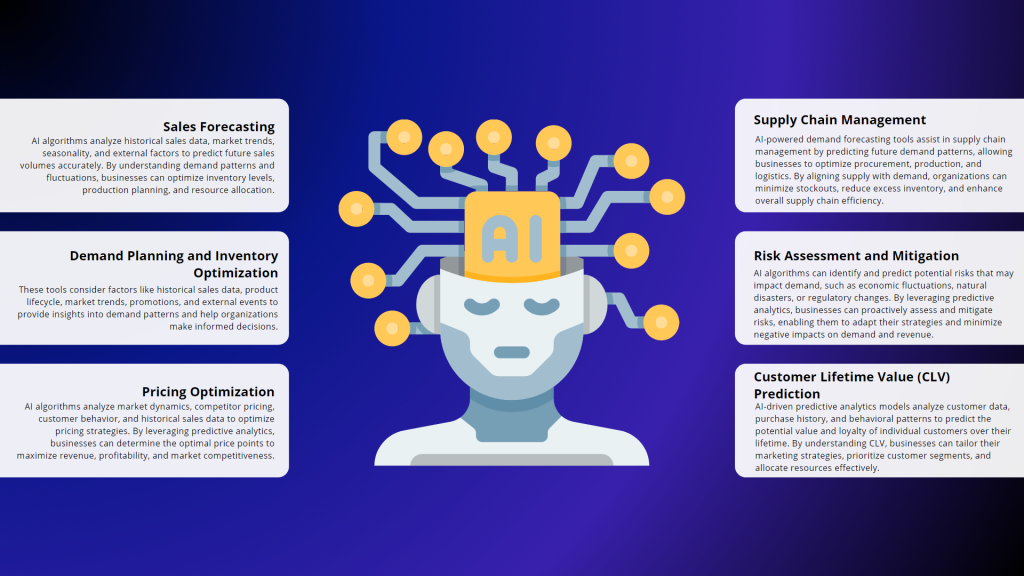
Introduction:
In the modern data-driven landscape, the ability to anticipate future trends and outcomes holds immense value for individuals, businesses, and organizations alike. Future forecasting enables us to make well-informed decisions, develop effective strategies, and proactively address potential challenges. Within this context, Artificial Intelligence (AI) has emerged as a powerful tool, revolutionizing the way we analyze data and make predictions. In this article, we will explore the concept of AI tools for future forecasting, their wide-ranging applications across diverse industries, and the techniques harnessed to unlock their potential.
- The Role of AI in Future Forecasting:
AI is a branch of computer science that empowers machines to emulate human intelligence and learn from experiences. By employing machine learning algorithms and predictive analytics, AI can efficiently process vast datasets, uncover hidden patterns, and discern insights that human analysts might overlook. This unique capability positions AI as an invaluable asset for future forecasting, leveraging historical data to predict upcoming trends and potential outcomes.
- Applications of AI in Future Forecasting:
AI’s impact spans numerous industries, fundamentally transforming decision-making processes. In the financial sector, AI-powered algorithms assess market trends and economic indicators, enabling predictions on stock prices and investment opportunities. In healthcare, AI tools facilitate disease outbreak predictions, patient outcome modeling, and drug discovery assistance. Supply chain management benefits from AI-driven demand forecasting and optimized inventory management. Additionally, AI-driven forecasting assists marketing and sales strategies by predicting customer behavior and preferences.
- Techniques for Future Forecasting Using AI:
a. Predictive Analytics: AI utilizes predictive analytics to analyze historical data, identify patterns, and establish relationships. Subsequently, this knowledge is applied to predict future events or behaviors.
b. Time Series Forecasting: Leveraging historical data, time series forecasting predicts future values based on patterns present in the time-dependent data.
c. Natural Language Processing (NLP): AI employs NLP to comprehend and analyze human language, extracting insights from unstructured data sources such as customer reviews, social media content, and news articles.
d. Simulation and Scenario Analysis: By creating simulations and conducting scenario analyses, AI facilitates the exploration of potential outcomes under various conditions, enabling decision-makers to prepare for multiple contingencies.
- Challenges and Ethical Considerations:
While AI tools offer great promise for future forecasting, certain challenges require careful consideration. Ensuring data quality, addressing bias, and enhancing the interpretability of AI-generated predictions are essential to maintain forecast accuracy and reliability. Moreover, ethical considerations, such as privacy and transparency, must be paramount to foster responsible AI deployment.
Conclusion:
AI tools for future forecasting are revolutionizing decision-making and strategic planning. By harnessing the capabilities of AI, businesses and organizations can gain a competitive edge, optimize resource allocation, and proactively prepare for the uncertainties of the future. As AI continues to advance, it will play an increasingly pivotal role in future forecasting, empowering us to navigate the complexities of an ever-evolving world with confidence.
Applications of AI in Future Forecasting
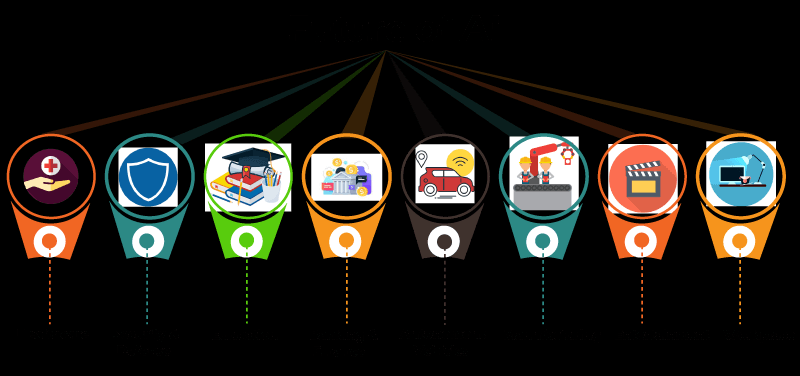
Artificial Intelligence (AI) has become a game-changer in future forecasting, offering a plethora of applications across various industries. By leveraging its data processing capabilities and predictive algorithms, AI empowers decision-makers to make informed choices and plan strategically. Let’s explore the diverse applications of AI in future forecasting:
- Financial Forecasting: AI algorithms analyze historical market data, economic indicators, and trading patterns to predict stock prices, market trends, and investment opportunities. Financial institutions use AI to optimize portfolio management and assess risks effectively.
- Demand Forecasting: AI-driven tools process historical sales data, customer behavior, and market trends to forecast demand for products and services. This aids businesses in optimizing inventory levels, production schedules, and supply chain operations.
- Sales and Revenue Prediction: AI analyzes past sales data, customer interactions, and marketing campaigns to forecast future sales and revenue. Organizations can set realistic targets and allocate resources efficiently.
- Weather Forecasting: AI techniques analyze vast weather datasets, satellite imagery, and atmospheric patterns to enhance weather forecasts and predict natural disasters more accurately.
- Healthcare Predictive Analytics: AI is used to predict patient outcomes, disease progression, and potential health risks by analyzing medical data. This assists in personalized treatment plans and proactive healthcare interventions.
- Marketing and Customer Behavior Analysis: AI analyzes customer data, online interactions, and social media engagements to predict customer preferences and behavior. This helps businesses tailor marketing strategies and improve customer experiences.
- Human Resources and Workforce Planning: AI aids in workforce forecasting by analyzing HR data, employee performance, and market trends. Organizations can predict talent requirements and plan recruitment strategies accordingly.
- Energy Demand Forecasting: AI is employed in the energy sector to predict energy demand based on historical usage patterns, weather conditions, and economic factors. This assists in efficient energy production and distribution.
- Transportation and Traffic Management: AI-powered algorithms analyze traffic data, historical patterns, and events to forecast transportation demand and traffic flow. This enables optimized public transportation and traffic management.
- Economic Forecasting: AI analyzes economic indicators, market data, and consumer behavior to predict economic trends and inform policy decisions.
- Sports Analytics: AI is utilized in sports to analyze player performance data, game scenarios, and historical trends to forecast match outcomes and optimize team strategies.
Conclusion:
The applications of AI in future forecasting are vast and diverse, empowering businesses and organizations with data-driven insights. AI’s ability to process vast amounts of data, recognize patterns, and predict outcomes revolutionizes decision-making and strategic planning across industries. As AI technology advances, its impact on future forecasting is poised to grow, enabling a more prepared and competitive approach to an ever-evolving world.
Techniques for Future Forecasting Using AI
Future forecasting using AI relies on a range of powerful techniques that harness machine learning algorithms and predictive analytics to analyze data and make informed predictions. These techniques enable businesses and organizations to anticipate trends, plan strategically, and make data-driven decisions. Here are some key AI techniques for future forecasting:
- Predictive Analytics:
Predictive analytics is a prominent AI technique used to forecast future events or behaviors. It involves analyzing historical data to identify patterns and relationships that can inform predictions. Machine learning algorithms, such as regression, decision trees, and neural networks, are commonly employed in predictive analytics to make accurate forecasts based on past patterns. - Time Series Forecasting:
Time series forecasting is a specialized technique used to predict future values based on historical time-dependent data. AI algorithms analyze patterns in past data and extrapolate them to predict future values. Time series forecasting is widely used in finance to predict stock prices, sales trends, and demand for products. - Natural Language Processing (NLP):
NLP enables AI systems to understand and analyze human language. In future forecasting, NLP plays a crucial role in extracting insights from unstructured data sources, such as customer reviews, social media posts, news articles, and surveys. It helps gauge public sentiment, assess customer feedback, and understand market trends for informed future predictions. - Simulation and Scenario Analysis:
Simulation and scenario analysis involve using AI to create simulations and explore potential outcomes under different conditions. Decision-makers can assess the impact of various strategies, external events, or market shifts on future outcomes. This technique aids in identifying optimal courses of action and preparing for different scenarios. - Clustering and Segmentation:
AI-powered clustering and segmentation techniques group similar data points based on specific attributes. This helps businesses identify distinct customer segments, market trends, or product categories, enabling tailored forecasting and targeted decision-making. - Ensemble Methods:
Ensemble methods combine multiple AI models to enhance forecasting accuracy. By aggregating predictions from different models, ensemble methods provide more robust and reliable future forecasts. - Anomaly Detection:
Anomaly detection using AI identifies unusual or abnormal patterns in data. In future forecasting, this technique helps detect unexpected events or outlier behaviors that may significantly impact outcomes. - Reinforcement Learning:
Reinforcement learning is used when forecasting involves decision-making in dynamic environments. AI models learn to take actions to maximize rewards or achieve specific goals over time. This technique finds application in areas such as supply chain management, where decisions need continuous adaptation based on changing circumstances.
Conclusion:
The techniques outlined above showcase the versatility of AI in future forecasting. By harnessing these powerful tools, businesses and organizations can gain valuable insights, make data-driven predictions, and prepare for various scenarios. AI’s capability to process vast amounts of data and identify complex patterns positions it as an indispensable asset for effective future forecasting across diverse industries.
Overcoming Challenges and Ethical Considerations
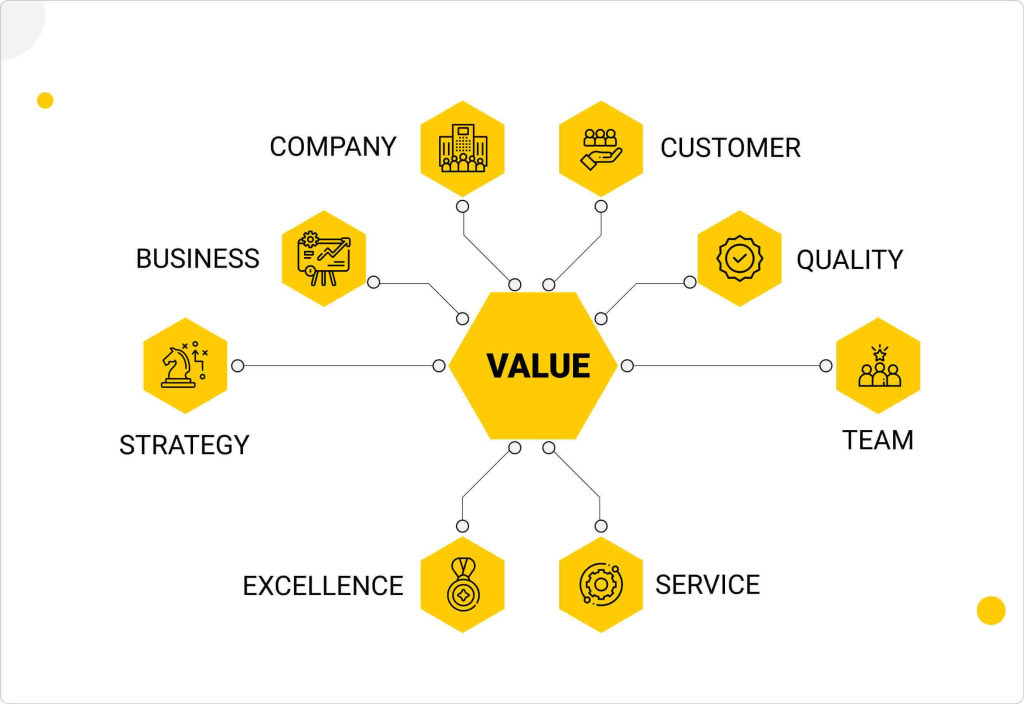
Overcoming Challenges and Ethical Considerations in Future Forecasting Using AI
As the utilization of AI in future forecasting becomes more prevalent, it presents numerous opportunities and challenges that necessitate thoughtful consideration to ensure ethical and responsible implementation. Below are key challenges and ethical considerations that must be addressed:
- Data Quality and Bias:
The accuracy of AI predictions heavily relies on high-quality data. Poor data quality or biased datasets can lead to inaccurate forecasts. Ensuring data integrity and diversity while mitigating biases is crucial to maintain reliable outcomes. - Interpretability and Explainability:
Certain AI models, such as deep learning neural networks, are often considered “black-box” systems, making it difficult to understand their decision-making process. To build trust and transparency, interpretable models and methods to explain AI-generated predictions are necessary. - Privacy Concerns:
AI-driven forecasting may involve handling sensitive data, raising privacy concerns. Safeguarding user information through data anonymization and adhering to privacy regulations is essential. - Overfitting and Generalization:
AI models can overfit to training data, resulting in poor generalization to new, unseen data. Rigorous testing and validation are required to ensure models are robust and generalize effectively. - Algorithmic Bias:
AI systems may perpetuate biases present in the training data, leading to unfair predictions. Addressing and mitigating algorithmic bias is imperative to ensure equitable outcomes. - Human Expertise:
Relying solely on AI for forecasting without human expertise may lead to misguided decisions. Combining AI-generated insights with human knowledge and judgment is crucial for accurate interpretation and validation. - Model Reliability and Uncertainty:
Assessing the reliability of AI models is vital to establish their accuracy and limitations. Communicating uncertainty and confidence intervals in forecasts allows decision-makers to make well-informed choices. - Long-Term Impact and Unintended Consequences:
Considering the potential long-term effects and unintended consequences of AI forecasting is essential to avoid adverse outcomes and ensure sustainability. - Ethical Use of AI:
Ethical considerations encompass using AI responsibly, promoting the well-being of society, and avoiding harmful or malicious applications. - Regulatory Compliance:
AI applications in future forecasting may be subject to industry-specific regulations. Ensuring compliance with relevant laws and guidelines is crucial to maintain ethical standards.
Conclusion:
Addressing challenges and ethical considerations is fundamental to harnessing the full potential of AI in future forecasting. Through transparency, fairness, and accountability, AI can be employed responsibly, enabling informed decisions that positively impact society and businesses alike. Adopting an ethical approach will be pivotal as AI continues to shape the future of forecasting.
Best Practices and Tips for Effective Future Forecasting with AI
Leveraging AI for future forecasting can provide organizations with valuable insights and a competitive edge. To ensure effective and accurate predictions, consider the following best practices and tips:
- Data Collection and Preparation:
Collect high-quality, diverse, and relevant data. Cleanse and preprocess the data to remove errors, outliers, and duplicates. Reliable forecasts depend on the quality of input data. - Clearly Define Objectives:
Establish clear and specific objectives for your future forecasting initiatives. Identify the questions AI should address and the key performance indicators (KPIs) to measure success. - Choose Appropriate AI Techniques:
Select AI techniques that align with your forecasting objectives. Techniques like predictive analytics, time series forecasting, and natural language processing may be suitable for different scenarios. - Regular Model Updating and Validation:
Continuously update AI models to remain relevant and accurate. Regularly validate models using historical data and compare their performance against actual outcomes. Fine-tune models as needed. - Combine AI with Human Expertise:
Integrate AI-generated insights with human knowledge and expertise. AI excels at processing data and identifying patterns, while human judgment provides context and decision-making capabilities. - Consider Uncertainty:
Acknowledge uncertainty in future forecasts. Provide confidence intervals or probabilistic forecasts to help decision-makers understand the range of possible outcomes. - Foster Collaboration:
Encourage collaboration between data scientists, domain experts, and decision-makers. Collaboration fosters a deeper understanding of business needs and ensures AI solutions align with real-world challenges. - Monitor and Track Performance:
Continuously monitor the performance of AI models and track their accuracy over time. This helps identify any deviations from expected results and facilitates model optimization. - Address Ethical Considerations:
Be mindful of ethical considerations, data privacy, and algorithmic bias. Implement strict privacy measures, identify and mitigate biases, and ensure responsible use of AI in future forecasting. - Agility and Adaptability:
Stay agile and adaptable in response to changing conditions. The future is dynamic, and assumptions may shift, so be prepared to adjust forecasting strategies and models accordingly. - Effective Communication:
Communicate the outcomes of AI-driven forecasts effectively to stakeholders. Use clear visualizations and explanations to help decision-makers understand and act upon the insights.
Conclusion:
By adhering to these best practices and tips, organizations can harness the full potential of AI for future forecasting. Effective data management, well-defined objectives, continuous validation, and collaboration between humans and machines are key to making accurate predictions and gaining a competitive advantage. Ethical considerations and clear communication further enhance the value of AI in shaping a promising future for businesses and society.
Thanks,
Leave a Reply
You must be logged in to post a comment.