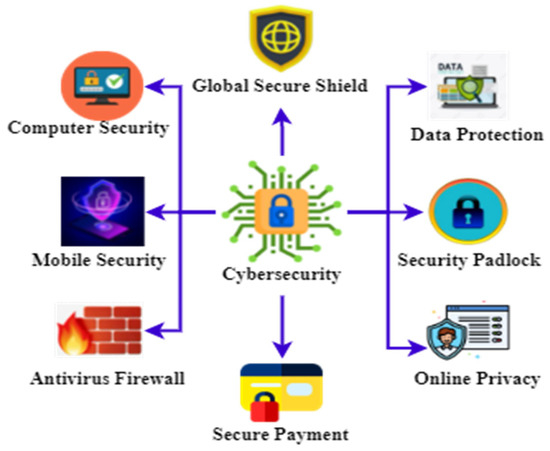
Introduction
In the rapidly evolving landscape of artificial intelligence (AI), the rise of narrow AI systems has sparked unprecedented advancements. These systems, also known as Weak AI, excel in specific tasks, from image recognition to language translation. However, amidst their numerous benefits, it is vital to acknowledge the potential threats that accompany their implementation. This article delves into the less-explored territory of the risks associated with narrow AI executions and emphasizes the need to mitigate these challenges.
- Data Bias and Discrimination
The foundation of narrow AI heavily rests on training data. Yet, if the data is biased or skewed, AI models may inadvertently perpetuate those biases. This can lead to discriminatory outcomes in areas such as hiring, lending, and criminal justice. The amplification of existing inequalities is a concern that necessitates addressing data bias comprehensively.
- Adversarial Attacks
Adversarial attacks involve manipulating input data to deceive AI systems. Minor adjustments to input information can cause AI models to produce incorrect outputs. For instance, altering an image slightly can lead to misinterpretations by an AI’s image recognition algorithms, potentially causing significant errors.
- Contextual Understanding Shortcomings
Narrow AI systems often lack contextual awareness and common sense reasoning. This limitation can lead to misinterpretations of human intentions or inappropriate responses. In critical situations, this deficiency could result in misunderstandings and unintended harmful actions.
- Dependency on Availability
Narrow AI’s functionality hinges on data access, connectivity, and server availability. In scenarios where these resources are disrupted, AI systems can fail. This dependency introduces vulnerabilities in applications that require real-time responsiveness or operate in remote environments.
- Unanticipated Consequences
Narrow AI systems are designed to excel in specific tasks, often sacrificing broader reasoning abilities. When faced with situations outside their training data, these systems might generate incorrect or unpredictable responses, leading to unintended and potentially adverse outcomes.
Addressing the Threats
- Data Quality and Bias Mitigation
Thoroughly reviewing and diversifying training data can help mitigate bias. Regular assessments of AI decision-making processes are crucial to detect and rectify discriminatory patterns.
- Robustness Testing
Conducting rigorous testing, including adversarial scenarios, can uncover vulnerabilities and enhance the resilience of AI models against potential attacks.
- Human Oversight and Intervention
Maintaining human oversight and the ability to intervene in AI decisions is imperative. Particularly in high-stakes situations, humans should have the final authority.
- Enhancing Contextual Awareness
Advancements in natural language processing and common-sense reasoning models can enhance AI’s comprehension of context, minimizing the risk of inappropriate responses.
- Ethical Frameworks and Regulations
Implementation of ethical guidelines and regulations for AI development and deployment ensures responsible and accountable AI practices.
Conclusion
As narrow AI systems integrate into various aspects of our lives and industries, understanding their inherent risks is paramount. While these systems hold remarkable potential, acknowledging their limitations and vulnerabilities is crucial. By employing strategies that address data bias, ensure robustness, maintain human oversight, and adhere to ethical frameworks, we can harness the capabilities of narrow AI while mitigating potential risks. Through responsible AI development, we can leverage the benefits of AI while upholding our values and security.
Thanks,
Leave a Reply
You must be logged in to post a comment.