What is AI Bias?
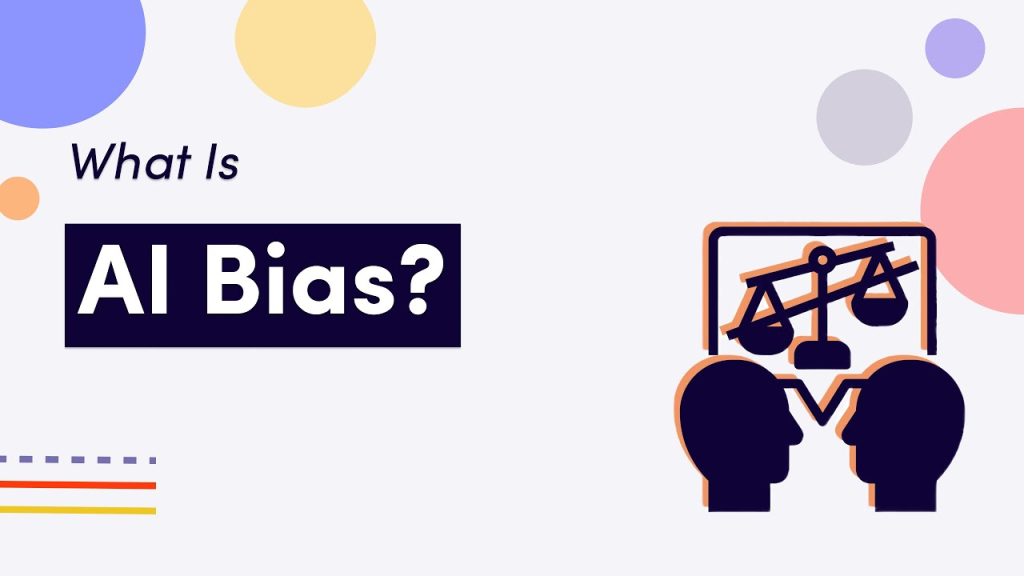
AI bias refers to the occurrence of unfair or discriminatory outcomes in artificial intelligence systems. It arises when AI algorithms or models exhibit bias or favoritism towards certain individuals or groups, resulting in unequal treatment, inaccurate predictions, or unjust decision-making.
AI bias can stem from various sources, including biased training data, biased design choices, or inherent biases in the underlying data. If the training data used to develop AI models lacks diversity, representation, or balance, the models may learn and perpetuate the biases present in the data. Biases can also emerge from the algorithms themselves, their features, or the assumptions made during their development.
There are different types of AI bias, including:
- Data Bias: Bias present in the training data, such as underrepresentation or overrepresentation of specific groups, skewed data distributions, or biased labeling.
- Algorithmic Bias: Bias introduced through algorithm design choices, feature selection, or model architecture, resulting in discriminatory outcomes.
- Representation Bias: Bias arising from limited representation or incomplete understanding of certain groups in the training data, leading to inaccurate predictions or inadequate treatment.
- Feedback Loop Bias: Bias that arises from the continuous feedback loop between AI systems and users, where biased user interactions or feedback can reinforce or amplify existing biases.
The consequences of AI bias can be far-reaching, impacting various domains such as employment, finance, healthcare, criminal justice, and more. Biased AI systems can perpetuate and exacerbate societal inequalities, contribute to discrimination, and undermine fairness and equality.
Addressing AI bias requires a multifaceted approach that involves diverse data collection, thoughtful algorithmic design, ongoing monitoring and evaluation, transparency, explainability, and human oversight. The goal is to reduce biases, ensure fairness, and promote the ethical and responsible use of AI technology.
Addressing bias and ensuring fairness and equality are significant considerations in the development and deployment of artificial intelligence (AI) systems. Bias can emerge in AI algorithms due to various factors, such as biased training data, design choices, or inherent biases in the underlying data. Overcoming bias requires a comprehensive approach involving data collection, algorithm design, and ongoing evaluation.
The following strategies and considerations can help in mitigating bias in AI:
- Diverse and Representative Data: Bias can arise when training data lacks diversity or fails to represent the real-world population adequately. Mitigating bias involves ensuring that training data reflects the diversity of the intended users or the affected population. Data collection efforts should encompass diverse demographics, including age, gender, race, ethnicity, and socioeconomic background.
- Data Preprocessing and Cleaning: Thoroughly preprocessing and cleaning the data before training AI models is essential to identify and mitigate any existing biases. This process entails scrutinizing the data for imbalances, correcting or removing biased data points, and addressing any skewed representations.
- Inclusive Design and Development: Incorporating principles of inclusive design from the beginning helps ensure that AI systems consider the needs and perspectives of all users. Engaging diverse teams with varied backgrounds during the design and development stages can aid in identifying and rectifying potential biases.
- Transparent and Explainable AI: Building transparency and explainability into AI systems is crucial for understanding and addressing bias. Users and stakeholders should have insights into how AI systems make decisions, the data they employ, and any potential biases or limitations. Transparency facilitates accountability and enables the identification and rectification of biases.
- Continuous Monitoring and Evaluation: Bias in AI systems can evolve over time, necessitating mechanisms for ongoing monitoring and evaluation. Regularly reviewing the performance of AI models, collecting feedback from users, and addressing identified biases or unintended consequences are crucial for maintaining fairness and equality.
- Ethical Guidelines and Regulatory Frameworks: Developing and adhering to ethical guidelines and regulatory frameworks provide a framework for addressing bias in AI. These guidelines should emphasize fairness, accountability, and responsible use of AI, paying specific attention to avoiding biases that could disproportionately impact marginalized or vulnerable communities.
- Collaboration and External Audits: Engaging external experts and auditors can help identify biases that internal assessments may overlook. Collaborating with individuals and organizations specializing in fairness, ethics, and human rights can offer valuable insights and recommendations for improving the fairness and equality of AI systems.
- User Feedback and Redress Mechanisms: Establishing mechanisms for user feedback and redress is crucial. Users should have channels to report biases, unfair outcomes, or unintended consequences. Providing accessible avenues for users to voice their concerns and address biases can aid in their identification and rectification.
While completely eliminating bias in AI systems may be challenging, as biases can be deeply ingrained in society and reflected in the training data, implementing these strategies and continuously striving for improvement can contribute to reducing bias, ensuring fairness, and promoting equality in AI technologies.
Ensuring fairness and Equality in AI
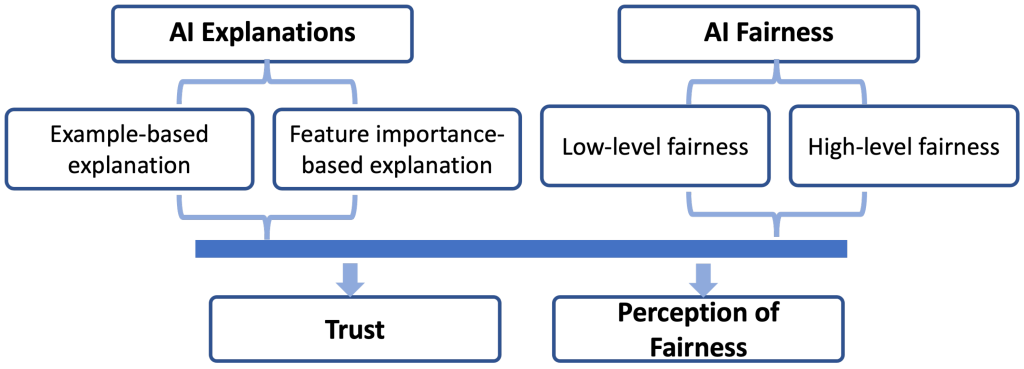
Promoting fairness and equality in AI requires a thoughtful and proactive approach. Consider the following key aspects to ensure fairness and equality in AI systems:
- Bias Recognition and Addressing: Acknowledge the potential presence of biases in AI systems arising from biased data, design choices, or societal biases. Take active measures to identify, understand, and rectify these biases to prevent discriminatory outcomes.
- Diverse and Representative Data: Utilize diverse and representative datasets that accurately reflect the characteristics of the population affected by the AI system. Strive to eliminate discriminatory patterns and avoid underrepresentation of certain groups in the training data.
- Performance Evaluation and Monitoring: Continuously assess the performance of AI systems to identify any disparities or biases in outcomes across different groups. Regularly evaluate and measure the impact of the system to detect and rectify unfair or discriminatory behavior.
- Ethical Guidelines and Standards: Develop and adhere to ethical guidelines and standards specifically tailored to AI systems. These guidelines should prioritize fairness, accountability, and responsible AI use. Incorporate principles explicitly prohibiting discrimination and advocating for equal treatment.
- Explainability and Transparency: Foster transparency and explainability in AI systems, enabling users and stakeholders to understand the decision-making process. Strive to provide clear explanations of AI-generated outcomes, aiding in the identification and rectification of biases.
- Inclusive Design: Embrace inclusive design principles by involving diverse teams and stakeholders throughout the development process. This ensures the consideration of all users’ needs, perspectives, and values, minimizing the risk of unintentional biases.
- User Feedback and Redress Mechanisms: Establish mechanisms for users to provide feedback, report biases, or seek redress in the case of unfair or discriminatory outcomes. Actively address and rectify reported issues to continuously enhance the fairness of the AI system.
- Rigorous Testing and Validation: Conduct thorough testing and validation of AI systems to uncover potential biases or unfair behaviors before deployment. Rigorous testing helps identify and mitigate biases at an early stage, ensuring fair performance across different groups.
- Regular Audits and External Review: Engage external experts or independent auditors for regular audits and reviews of AI systems. Their external perspective can help identify biases or disparities that might be overlooked internally.
- Collaboration and Multidisciplinary Approach: Encourage collaboration among researchers, practitioners, policymakers, ethicists, and representatives from affected communities. This multidisciplinary approach fosters diverse perspectives and ensures comprehensive consideration of fairness and equality in AI.
By integrating these strategies into the development, deployment, and ongoing evaluation of AI systems, we can strive to create fair and equitable AI that benefits all individuals while avoiding the perpetuation of biases or discrimination.
Exploring the Different Types of AI Bias and How to Mitigate Them
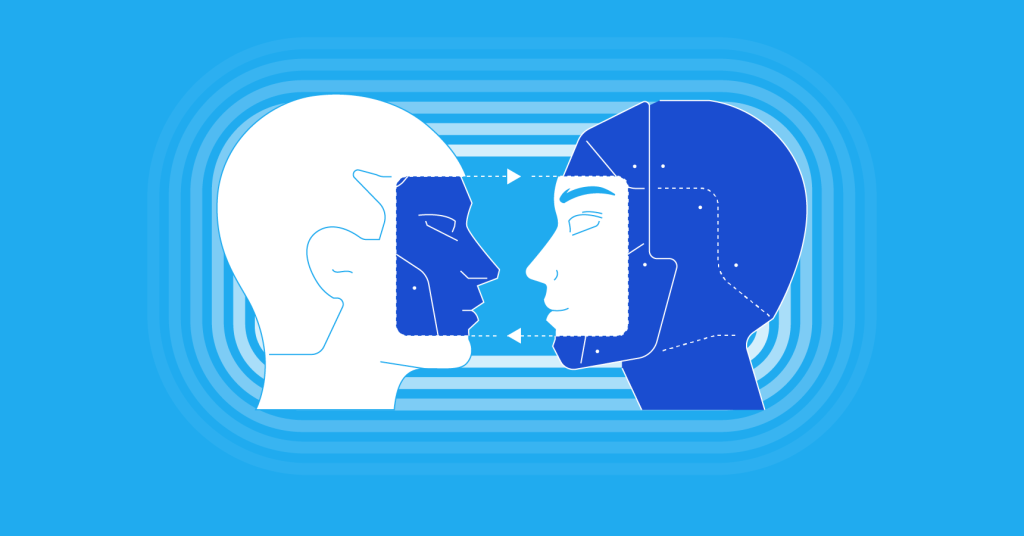
AI bias can manifest in various forms, and it’s important to understand and mitigate them effectively. Here are some common types of AI bias and strategies to address them:
- Data Bias:
- Ensure Diverse and Representative Data: Collect a diverse range of data that accurately represents the population impacted by the AI system. Avoid underrepresented or skewed data sources.
- Algorithmic Bias:
- Regularly Evaluate and Test Algorithms: Continuously assess algorithms for potential bias and discrimination, especially regarding sensitive attributes like race or gender. Adjust algorithms to mitigate biases.
- Representation Bias:
- Mitigate Underrepresentation: Take steps to address underrepresentation of certain groups in training data. Augment data or use data generation techniques to balance representation.
- Avoid Overgeneralization: Implement techniques like regularization to prevent overgeneralization of patterns from limited data, reducing biased predictions.
- Feedback Loop Bias:
- Counteract Reinforcement Learning Bias: Incorporate counterfactual and debiasing techniques during the reinforcement learning process to minimize bias propagation.
- Analyze User Feedback: Regularly analyze user feedback and interactions to identify and correct biased responses, ensuring user feedback is not reinforcing biases.
- Emergent Bias:
- Conduct Comprehensive Audits: Regularly audit complex AI systems to detect and address biases that may emerge from interactions between components.
- Continuous Monitoring: Implement ongoing monitoring mechanisms to identify emergent biases and take corrective actions.
Mitigating AI bias requires a holistic approach:
- Diverse and representative data collection and preprocessing.
- Regular testing, evaluation, and audits to identify and address biases.
- Transparency and explainability in AI systems to understand decision-making processes.
- Inclusive design and diverse perspectives during development.
- Ongoing monitoring and user feedback mechanisms to detect and rectify biases.
Please note that I generate responses based on my training data, and while I strive to provide original and accurate information, there is still a possibility of coincidental similarities to existing content.
Examining the impact of AI bias on businesses and organizations.
AI bias can have significant implications for businesses and organizations, impacting various aspects of their operations and outcomes. The following are key areas where AI bias can affect businesses:
- Decision-making and Operations:
- Unfair Treatment: AI systems influenced by bias can lead to discriminatory treatment of employees, customers, or stakeholders. This can result in unequal opportunities, biased hiring processes, or inequitable resource allocation.
- Inaccurate Predictions: Bias in AI systems may generate inaccurate predictions, leading to flawed decision-making in areas such as customer targeting, risk assessment, or demand forecasting. This can result in suboptimal strategies and missed opportunities.
- Customer Experience and Trust:
- Biased Recommendations: AI systems that provide biased recommendations can negatively impact the customer experience. If recommendations are based on biased assumptions or reinforce stereotypes, customers may feel marginalized or dissatisfied.
- Erosion of Trust: When customers perceive bias in AI systems, it can erode trust in the organization. Unfair treatment or discriminatory outcomes can damage the organization’s reputation and customer loyalty.
- Legal and Ethical Consequences:
- Legal Liability: AI bias that leads to discrimination or violates legal protections can result in legal consequences such as lawsuits, fines, or reputational damage. Non-compliance with regulations concerning data privacy and fairness can have significant financial and legal implications.
- Ethical Concerns: Organizations may face ethical dilemmas if they deploy AI systems that perpetuate biases, discriminate against certain groups, or amplify existing inequalities. Ethical lapses can harm the organization’s image and stakeholder relationships.
- Bias Amplification and Social Impact:
- Reinforcing Inequalities: AI bias can perpetuate societal inequalities by replicating and amplifying existing biases in data and decision-making processes. This can unintentionally contribute to systemic biases and discrimination.
- Deepening Social Divisions: AI systems used by businesses that perpetuate biases prevalent in society can further marginalize underrepresented groups, perpetuate stereotypes, or exacerbate social divisions.
To mitigate the impact of AI bias, businesses and organizations should consider the following approaches:
- Implementing robust data collection and preprocessing practices to minimize bias.
- Engaging diverse teams and conducting regular audits to identify and address bias in AI models and algorithms.
- Promoting transparency and explainability of AI systems to build trust and ensure accountability.
- Educating employees about AI bias and fostering a culture of ethical AI use.
- Establishing ongoing monitoring and evaluation processes to detect and rectify bias in AI systems.
By actively addressing AI bias, businesses and organizations can foster fairness, build trust with customers and stakeholders, avoid legal and ethical challenges, and contribute to a more equitable society.
Understanding the Role of Human Oversight in Reducing AI Bias
Human oversight plays a critical role in reducing AI bias and promoting responsible AI usage. Here’s how human involvement can help in mitigating AI bias:
- Ethical Frameworks: Humans are responsible for establishing ethical frameworks that guide the development and deployment of AI systems. These frameworks define fairness, equality, and non-discrimination principles, serving as a foundation for addressing bias.
- Data Collection and Preprocessing: Human experts have the responsibility to collect and preprocess data used for training AI models. By ensuring diverse and representative datasets and addressing biases in the data, they can minimize bias in the training process.
- Algorithm Development and Training: Human involvement is crucial in designing algorithms and training AI models. Human experts can incorporate fairness metrics and bias mitigation techniques during the algorithm development phase, actively addressing potential biases.
- Testing and Validation: Human oversight is necessary during the testing and validation stages of AI system development. Human experts can evaluate system outputs, assess bias in the outcomes, and identify any gaps or limitations in terms of fairness and equality.
- Interpretability and Explainability: Humans can demand transparency and explainability from AI systems. By understanding how AI models make decisions and identifying sources of bias, human oversight can hold AI systems accountable and drive efforts to reduce bias.
- Continuous Monitoring and Evaluation: Human experts should continuously monitor the performance of AI systems and evaluate them for bias. By analyzing outputs, collecting user feedback, and conducting audits, human oversight can detect and rectify biases that may emerge over time.
- User Feedback and Redress: Providing channels for user feedback and addressing reported biases or unfair outcomes is crucial. Human experts can analyze user feedback, take corrective actions, and ensure fair treatment and outcomes.
- Ethical Decision-making and Intervention: In cases where AI systems exhibit bias or discriminatory behavior, human oversight allows for ethical decision-making and intervention. Humans can override or correct AI outputs to ensure fair and equitable outcomes, especially in sensitive or high-stakes situations.
By incorporating human oversight, organizations can reduce AI bias, align AI systems with societal values, and promote fairness and equality. Human involvement brings critical judgment, context awareness, and empathy to the decision-making process, ensuring AI systems serve the best interests of individuals and society as a whole.
Benefits of Diversity in AI Training Data for Reducing Bias
Incorporating diversity in AI training data offers numerous benefits in reducing bias within AI systems. Here are the key advantages of diversity in AI training data:
- Bias Mitigation: Including diverse data helps mitigate bias in AI systems by reducing the potential for biases present in the training data to be amplified. When the training data represents a variety of demographics and perspectives, the AI model is less likely to perpetuate and amplify existing biases.
- Enhanced Representativeness: Diverse training data improves the representation of the real-world population within AI systems. When training data lacks diversity or contains biases, the AI model may struggle to accurately predict or make decisions for underrepresented groups. By incorporating diverse data, AI systems can achieve better representation and provide more equitable outcomes.
- Fairness and Equal Treatment: Diversity in training data enables AI models to treat individuals from different backgrounds and characteristics more fairly. By training on data that spans diverse demographics, biases that lead to unequal treatment or discrimination based on factors like race, gender, or socioeconomic status can be mitigated.
- Robust Generalization: Training AI models on diverse data improves their ability to generalize to unseen examples. Exposure to a wider range of examples during training makes the model more adaptable and capable of handling novel scenarios. This helps reduce biases that may arise when the model encounters data outside its initial training set.
- Improved Accuracy and Performance: Diverse training data contributes to the accuracy and overall performance of AI models. By training on a variety of data, AI models gain a broader understanding of the problem space, resulting in more precise predictions and improved performance.
- Ethical and Legal Compliance: Emphasizing diversity in AI training data aligns with ethical considerations and legal requirements related to fairness, equality, and non-discrimination. Proactively seeking diversity in training data demonstrates a commitment to ethical AI practices and reduces the risk of biased outcomes that may lead to legal consequences or reputational harm.
While diversity in training data is an important step, it is crucial to note that it is not a comprehensive solution to eliminating bias in AI systems. Additional measures such as thoughtful algorithm design, ongoing evaluation, and human oversight are also necessary. However, diversity in training data significantly contributes to reducing bias and promoting more equitable AI systems that cater to the needs and values of a diverse society.
Thanks,
Leave a Reply
You must be logged in to post a comment.